On Friday, August 5, Standard & Poor's downgraded the credit rating of the U.S. long-term debt to AA+. On Monday, the first day the markets opened since the downgrade, the Dow Jones Industrial average dropped 5.6 percent and the S&P 500 fell 6.7 percent — the biggest single day drops since the crisis in 2008. A lot of people might be confused about this turmoil in the markets, since US debt is still considered one of the safest investments there is. Jay Forrester, founder of the field of System Dynamics, calls puzzles like this the "counterintuitive behavior of social systems."
Undoubtedly, the world economy is incredibly complex, and no individual or organization has a complete picture of how it works or where it's headed. Through pricing, the market is supposed to aggregate all of the pieces of partial information that we each hold and then converge to the "truth" — that is prices should reflect true underlying value. In some situations this can actually work. Prediction markets have been shown to be valuable tools for businesses to harvest the "wisdom of the crowds" and assess the probabilities that future events occur. But, this mechanism works best when individuals place their trades independently based on their own private information. In the real world, market dynamics are fundamentally social dynamics and as such they are subject to cascades of panic and the accumulation of overconfidence (what Alan Greenspan famously referred to as "irrational exuberance" (see also Robert Shiller)).
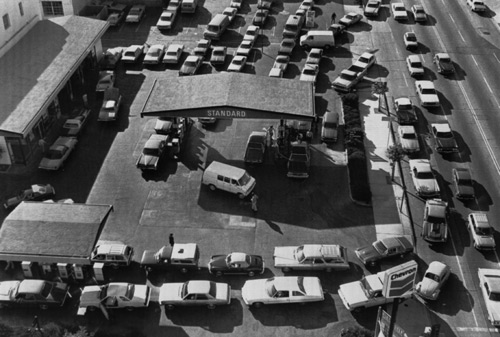
The current panic illustrates how even when there is no fundamental basis for a panic, social dynamics can amplify the signal of a panic to the point where an actual crisis ensues. The gas shortages of 1979 are a classic example of this phenomenon. The Iranian revolution sharply cut oil imports to the US from Iran. Nervous consumers rushed to top off their tanks and even to hoard gasoline at home. This drained the supply of gasoline at filling stations leading to an actual gasoline shortage. Word-of-mouth and media coverage reinforced consumer fears of shortages, leading to even more topping off and hoarding, as well as government policies such as odd/even day purchase rules that actually further incentivized consumers to top off frequently and store gasoline at home. Surprisingly, despite the very real shortage of gasoline at filling stations, US oil imports for the year actually increased in 1979 compared 1978. The crisis was caused by social dynamics, not an actual drop in supply. (See Sterman, Business Dynamics p. 212).
A similar but more comical crisis occurred in 1973 when Johnny Carson made a joke saying, "You know what’s disappearing from the supermarket shelves? Toilet paper. There’s an acute shortage of toilet paper in the United States." Consumers rushed out to stock up on toilet paper, leading to a real toilet paper shortage in the US that lasted several days. Even though Carson tried to correct the joke a few days later, by that time toilet paper was in fact in short supply because people were hoarding it at home.